Executive Summary
This paper aims to focus on Macmillan Cancer Support’s big data analysis and potential improvements that would allow it to ensure effective decision-making. Currently, this organization lacks a clearly defined vision related to big data usage, which is caused by insufficient design development and paying little attention to analytics. Accordingly, big data analysis is proposed as the decision to enhance its operational efficiency by analyzing patient satisfaction.
Data Gap Analysis
Data gap analysis involves evaluating the present data of an organization. It helps in gauging the performance of companies and evaluating the data that would greatly benefit them. For example, Apple Inc. might do data gap analytics to identify the areas where the consumers need the product to be changed. A good consideration is the Macmillan Cancer Support organization. It has over 1,474 working staff and over 6.7 million people affected as its members (Facts & figures, 2020). Macmillan Cancer Support organization uses different sources of data to support people living with cancer.
This data is used to enlighten good decision-making and to be clear on how cancer data is collected. According to Brzychczy and Liebetrau (2020), the United Kingdom has almost 3 million people living with cancer. Their research predicts that this figure will upsurge to 3.5 million by 2025 (Brzychczy and Liebetrau, 2020). Considering the gravity of the statistics, it is clear that data gap analysis measures should be taken to ensure that most people are not affected by cancer. In addition, the analytics will help in helping the current patients with better healthcare.
The cancer patient experience survey (CPES) is used to provide data. In this case, the results of CPES are used to understand better how patients feel about the care they are receiving. It is also used to identify how their bodies respond to prescriptions and the improvements to be made. Local cancer intelligence also works with partners to develop online tools. They have the right technological policies to protect data which helps them maintain their reputation (Grover and Kar, 2017, p. 223).
Improvements to Organizational Data
Macmillan Cancer Support works in partnership with the National Cancer Registration to ensure better and improved services. Hence, this helps in ensuring there is no data analyzing gap in their organizations. Data integrity is observed by ensuring that only authorized personnel has access to the data. Moreover, the data is securely encrypted to avoid any possibility of breach of patients’ information if the data lands in the wrong hands. Potential gaps in data analytics include unclear lifestyle habits of patients and reluctance to provide family medical history.
Roadmap to Development or Enhancement of the Big Data Infrastructure
All health organizations need a clear and simple data analytics process and tools. Macmillan Cancer Support is undergoing increasing expenses and can no longer give in to modest agreement tenders. The first milestone of the proposed roadmap is to define patients’ concerns by starting with the accurate and correct queries (Ha et al., 2018, p. 9). The second step is to design big data architecture, considering the needs of the organization. Third, it is possible to recommend establishing vibrant dimension priorities (Johanes and Thille, 2019). Macmillan Cancer Support should choose the statistics needed to answer crucial queries. In this case, they will need to know the amount of staff and their working hours. Fourth, thinking about how to measure the collected data and design is important. The work on data quality improvement should be based on a series of pilot studies and test implementations. Ultimately, the users of bid data should be educated to integrate it into their practice.
Data Collection
After defining the questions clearly and measuring priorities, the following should be considered: the file storing and the naming system. It helps to save time and avoid collecting the same information more than once. One should develop an interview template ahead of time if one needs to collect data through observation and interviews (Khalid, Sharma, and Dubey, 2019, p. 43). Data should be manipulated in different ways, such as creating a pivot table in excel, which helps better answer questions.
In addition, the attained results should be interpreted. The organization should question them the following issues: does the statistics answer the original query, and how does it respond to it? Are there any restrictions on your inferences or any area you ought to put into consideration? (Koechlin, 2020). If an organization’s interpretation answers those questions, it is clear that they have come to a productive conclusion.
Using Proposed Big Data Analytics in Organizational Decision-Making
Data analytics has been used for a long time as a valuable way for businesses to improve their decision-making strategy (Koechlin, 2020, p. 4). It helps in improving financial decisions as decisions on how to manage cash flow and get assets are of major importance. Secondly, strategic decisions can also be improved through big analyzing bid data (Marchau et al., 2019). Furthermore, operational business decisions require analyzed data for better performance. This includes decisions on how to market goods and services from the organization.
Additionally, workforce decisions benefit a lot for large data analytics. There must be a criterion on hiring staff that is competent, choosing proper methods of approaching them. Lastly, large data analytics helps the organization with structural decision-making (Tonidandel, King, and Cortina, 2018). Big data analytic allows simplifying the organizational structure. In this case, an operational business design and control process can be applied (Figure 1).
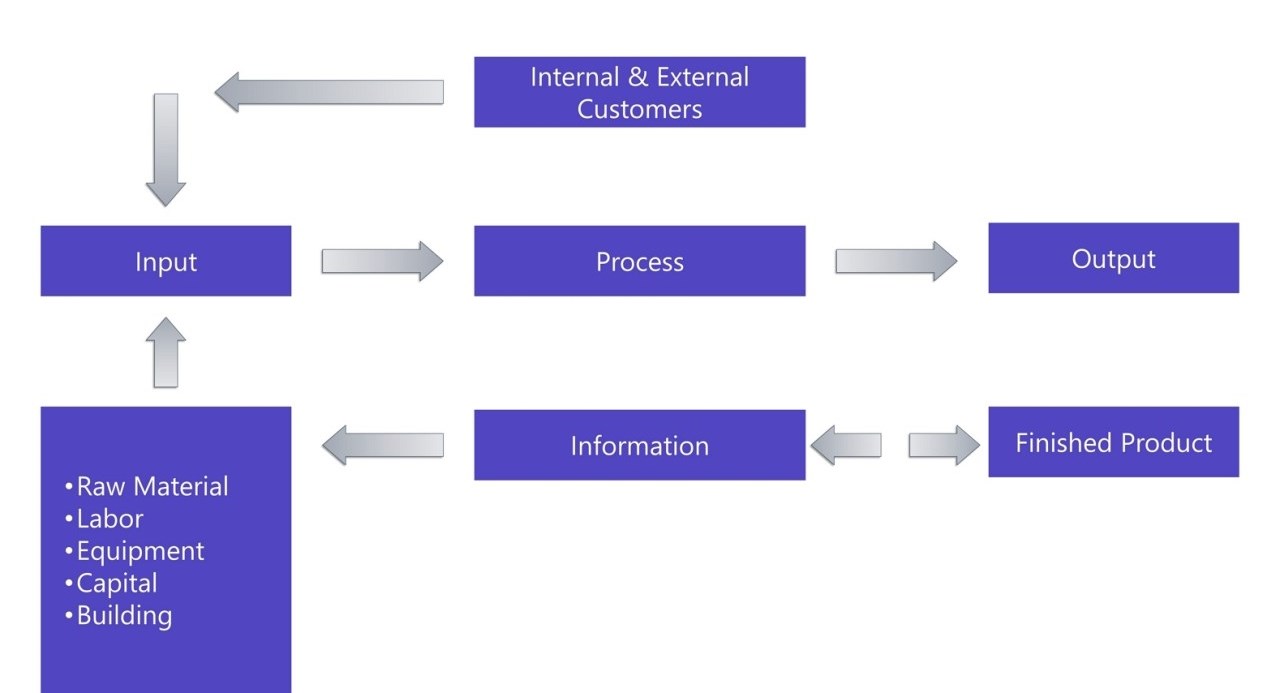
Operational decisions can be modeled once and reused several times (for example, calculating hospital bills for a patient) (Viqueira et al., 2020). In Macmillan Cancer Support, big data analytic should be used to improve operational efficiency through analyzing patient satisfaction using their attendance, medical, and perception data. It is the question to be solved since currently, the organization lacks a proper decision-making strategy to make sure that patients with cancer receive relevant services.
Reference List
Brzychczy, P. and Liebetrau, M. (2020) ‘Data analytic approaches for mining process improvement — machinery utilization use case’, Resources, 9(2), pp. 1-17.
Facts & figures. (2020) Web.
Grover, P. and Kar, A.K. (2017) ‘Big data analytics: a review on theoretical contributions and tools used in literature’, Global Journal of Flexible Systems Management, 18(3), pp. 203-229.
Ha, M. K. et al. (2018) ‘Toxicity classification of oxide nanomaterials: effects of data gap filling and PChem score-based screening approaches’, Scientific Reports, 8(1), pp. 1-11.
Johanes, P. and Thille, C. (2019) ‘The heart of educational data infrastructures – conscious humanity and scientific responsibility, not infinite data and limitless experimentation’, British Journal of Educational Technology, 50(6), pp.2959-2973.
Khalid, A.M., Sharma, S. and Dubey, A.K. (2019) ‘Data gap analysis, indicator selection, and index development: a case for developing economies’, Social Indicators Research, 4(1), pp. 1-68.
Koechlin, E. (2020) ‘Human decision-making beyond the rational decision theory’, Trends in Cognitive Sciences, 24(1), pp. 4-6.
Kolajo, T., Daramola, O. and Adebiyi, A. (2019) ‘Big data stream analysis: a systematic literature review’, Journal of Big Data, 6(1), pp. 1-30.
Marchau, V.A., et al. (2019) Decision making under deep uncertainty: from theory to practice. New York: Springer.
Tonidandel , S., King, E.B. and Cortina, J.M., (2018) ‘Big data methods: leveraging modern data analytic techniques to build organizational science’, Organizational Research Methods, 21(3), pp. 525-547.
Viqueira, J. R. et al. (2020) ‘Smart environmental data infrastructures: bridging the gap between earth sciences and citizens’, Applied Sciences, 10(3), pp.856-888.